For this reason, insurance companies should prioritise assessing their data and actively work to enhance its quality before delving into the realm of automation.
The following stages outline a structured process for insurers to follow before immersing themselves in automation. This thoughtful approach will prevent potential shortcomings and maximise returns on investment.
Steps for Enhancing Data Quality
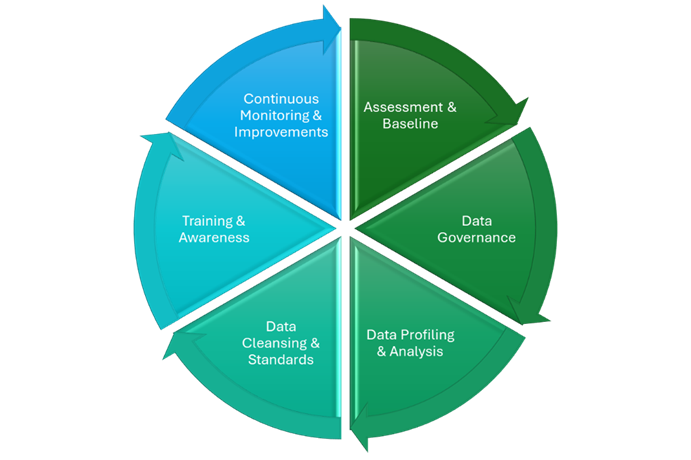
- Assessment and Baseline Establishment:
- Data Audit: Conduct a thorough audit of existing data to identify issues, inconsistencies, and gaps.
- Define Metrics: Establish KPIs and metrics to measure data quality.
- Baseline Assessment: Establish a baseline data quality level against which to measure improvements.
- Data Governance Implementation:
- Policy Development: Formulate data quality policies and standards.
- Governance Structure: Define roles and responsibilities for data ownership and stewardship.
- Communication: Communicate data quality expectations across the organisation
- Data Profiling and Analysis:
- Profiling: Use data profiling tools to analyse the structure, content, and quality of data.
- Identify Anomalies: Detect anomalies, errors, and inconsistencies within the datasets.
- Prioritise Issues: Prioritise data quality issues based on their impact on business processes.
- Data Cleansing and Standardisation:
- Cleanse Data: Implement automated tools to correct errors and inconsistencies.
- Standardisation: Standardise data formats, units, and naming conventions.
- Enforce Validation Rules: Set up validation checks to ensure data conforms to predefined rules.
- Training and Awareness:
- Employee Training: Conduct training programmes to educate employees on the importance of data quality.
- Promote Ownership: Encourage a culture of data ownership and responsibility.
- Feedback Mechanism: Establish a feedback mechanism for employees to report data quality issues.
- Continuous Monitoring and Improvements:
- Real-time Monitoring: Implement real-time or near-real-time monitoring of data quality.
- Feedback Loops: Create feedback loops for continuous improvement based on monitoring results.
- Iterative Process: Iterate on data quality processes based on feedback and evolving business needs.
By following these stages, organisations can establish a structured and iterative approach to improving data quality, ensuring that data is reliable, accurate, and aligned with business objectives.
For more information contact here.